Exploring the Role of AI in Tackling Climate Change
1. Understanding the Basics of AI and Its Capabilities.
AI leverages algorithms and advanced computational models to analyze vast datasets. This ability allows AI to identify patterns, draw insights, and predict outcomes with high precision.
Machine learning (ML), a subset of AI, uses historical data to improve its predictions over time without explicit programming.
AI’s capabilities extend to recognizing trends in climate data, optimizing energy consumption, and predicting natural disasters.
Advanced neural networks, for instance, can simulate complex environmental systems. They correct deviations between predicted and observed data, enhancing the accuracy of climate models.
Furthermore, AI-infused sensors monitor real-time environmental parameters like:
- temperature
- humidity
- pollution levels
Contributing valuable data that conventional methods might miss.
2. Linking AI With Environmental Science
AI intersects with environmental science to enhance understanding and predictive modeling. Machine learning algorithms process vast climate-related datasets, recognizing subtle correlations.
This information feeds into climate models, refining their predictive power.
For example, AI platforms analyze satellite imagery to measure deforestation rates, glacier movements, and ocean temperatures.
These insights help scientists gauge the health of ecosystems and devise effective conservation measures.
AI also aids in climate mitigation strategies. Predictive analytics optimize energy grids, integrating renewable sources seamlessly.
AI models forecast energy demands, reducing wastage and enhancing efficiency.
Even in agriculture, AI-driven tools suggest optimal irrigation schedules based on weather patterns.
By combining AI with environmental science, more accurate and actionable data informs policy decisions and climate solutions.
AI Applications in Climate Prediction
Weather Forecasting Enhancement
AI transforms weather forecasting by analyzing colossal amounts of data from satellites, sensors, and historical climate records. Machine learning models improve the accuracy of weather forecasts.
For example, the European Centre for Medium-Range Weather Forecasts (ECMWF) integrates AI techniques to enhance numerical weather prediction models significantly.
This leads to more:
- precise short-term
- long-term forecasts
- aiding in critical decision-making for agriculture
- aviation
- disaster preparedness
Predictive Analytics for Climate Patterns
AI-driven predictive analytics identify long-term climate patterns by processing complex datasets, including atmospheric conditions and oceanic currents.
The World Meteorological Organization (WMO) leverages AI to forecast phenomena such as El Niño and La Niña, which significantly affect global weather patterns and agricultural productivity.
By understanding these predictions, policymakers can implement strategies to mitigate adverse impacts on vulnerable regions.
This ensures that communities globally can prepare more effectively for climate-related challenges like droughts, floods, and heatwaves.
AI in Climate Change Mitigation
Reducing Carbon Footprints Through Smart Infrastructure
Investing in smart infrastructure reduces carbon footprints by improving energy efficiency.
AI algorithms monitor real-time data from buildings and utility systems to optimize energy usage.
For example, AI systems control heating, ventilation, and air conditioning (HVAC) systems by adjusting settings based on occupancy patterns and weather conditions.
Google’s DeepMind AI reduced the energy used for cooling its data centers by 40% through similar methods.
AI also enhances the effectiveness of renewable energy sources. Smart grids, leveraging AI, predict energy demand and adjust the supply from renewable sources accordingly.
This reduces reliance on fossil fuels and minimizes greenhouse gas emissions.
For example, AI-driven wind farms optimize turbine performance by analyzing wind patterns and adjusting blade angles in real-time.
AI-Driven Resource Management and Energy Optimization
Resource management becomes more efficient with AI-driven solutions. AI analyzes vast datasets to identify inefficiencies in water, waste, and energy systems.
For instance, AI-powered sensors detect water leaks in municipal systems, reducing water waste and conserving this vital resource.
In the energy sector, AI optimizes energy distribution and consumption.
AI-driven predictive maintenance identifies potential failures in energy systems before they occur, minimizing downtime and ensuring efficient operation.
For example, AI systems monitor and manage electricity grids, predicting peak demand periods and optimizing the distribution to avoid blackouts.
AI’s role in climate change mitigation extends to agriculture by optimizing resource use.
Precision farming, powered by AI, uses data from sensors, satellite imagery, and weather forecasts to provide farmers with insights on the optimal use of water, fertilizers, and pesticides.
This not only enhances crop yields but also reduces the environmental impact of farming practices.
Together, these AI-driven initiatives significantly contribute to reducing environmental impacts and advancing climate change mitigation efforts.
Challenges and Limitations
Ethical Concerns and AI Bias
When I analyze AI’s role in climate change, ethical concerns and biases present significant challenges. Algorithms, if trained on biased data, can lead to skewed results, affecting climate models and predictions.
For instance, datasets lacking diverse global data may miss regional climate variations. This bias limits AI’s capacity to provide accurate and equitable predictions across different geographies.
Furthermore, ethical considerations arise when deploying AI in vulnerable communities, where improper implementation could exacerbate existing inequalities.
An example is the potential for AI systems to prioritize data-rich regions, leaving underrepresented areas out of critical climate initiatives.
The Sustainability of AI Itself
Evaluating the sustainability of AI itself reveals another limitation. AI technologies, particularly machine learning models, demand substantial computational resources, which consume significant energy.
Training these models can lead to high carbon emissions, contradicting climate mitigation efforts. For example, training a single large AI model can emit as much carbon as five cars over their lifetimes.
This creates a paradox where AI, designed to combat climate change, might contribute to it. Solutions include optimizing algorithms for energy efficiency and increasing reliance on renewable energy sources for data centers.
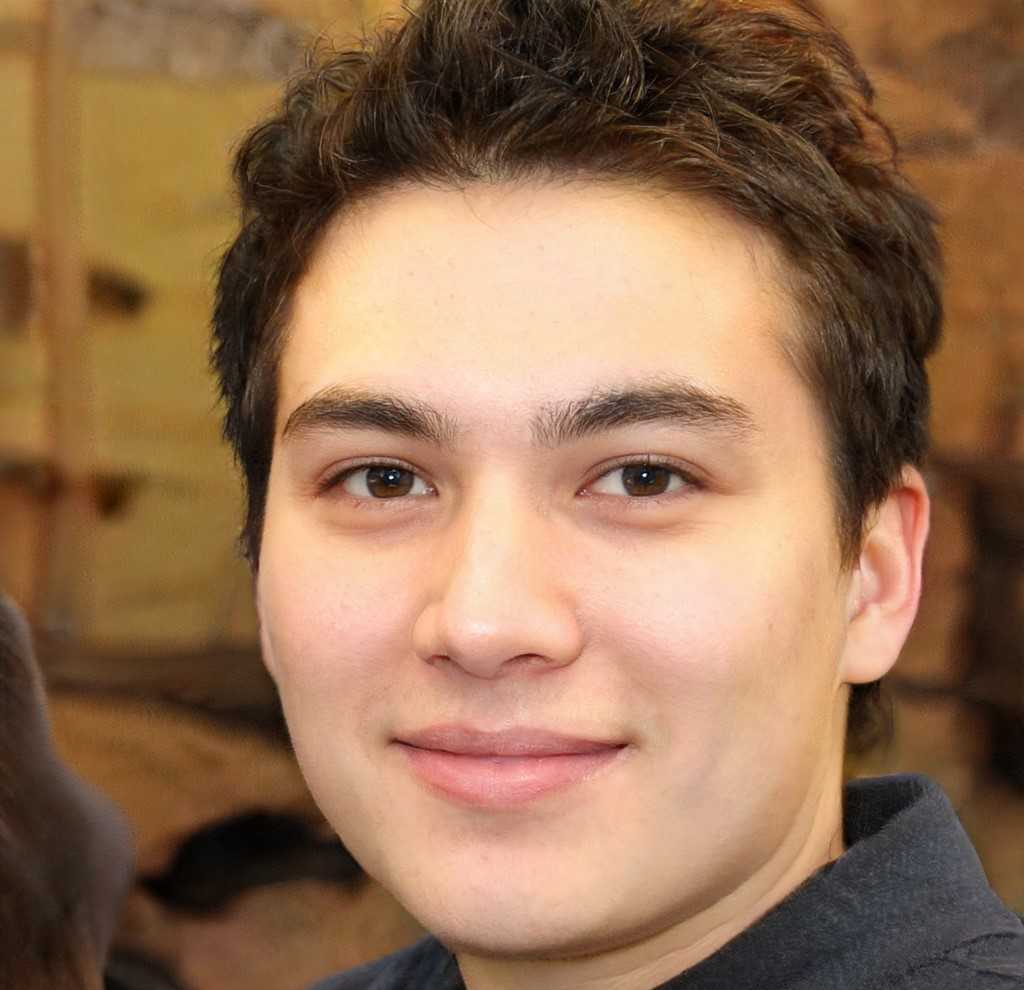
About the author:
Gerthann Stalcupy, the founder of your gtech colony , plays a pivotal role in shaping the direction and content of the platform. As the visionary behind the site. – Learn more