Understanding Artificial General Intelligence (AGI)
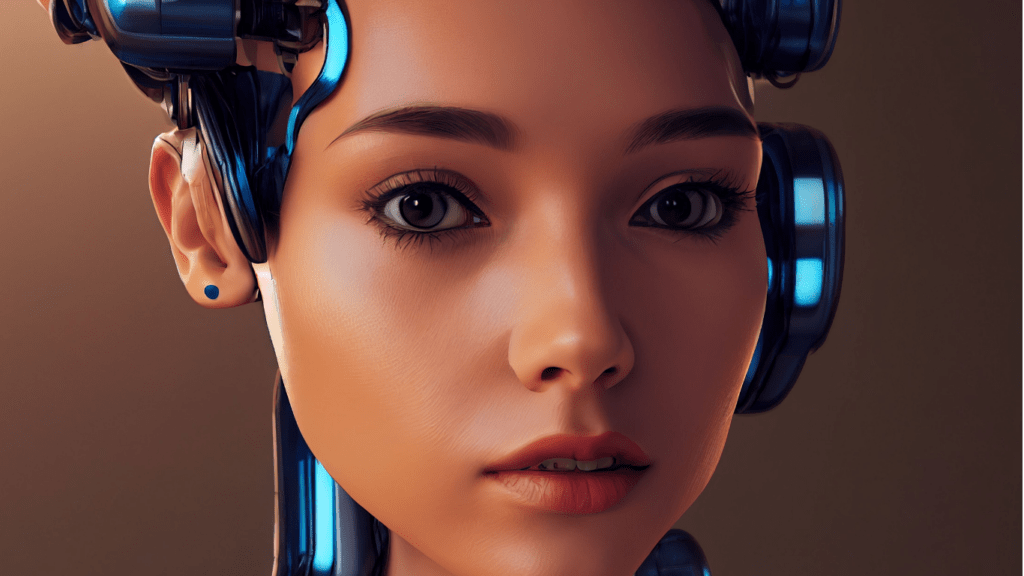
What Is AGI?
Artificial General Intelligence (AGI) refers to a type of artificial intelligence that can mimic human cognitive abilities across various tasks.
Unlike specialized AI systems designed for specific functions like language translation or image recognition, AGI aims to perform any intellectual task that a human can do.
This broad capability includes reasoning, problem-solving, learning, and understanding natural language.
AGI holds the promise of transforming multiple industries by automating complex decision-making processes, offering powerful new tools for research and development, and providing personalized healthcare solutions.
It stands as a significant milestone in AI research, striving to achieve a level of versatility and flexibility akin to human intelligence.
Key Differences Between AGI and AI
AI, or Artificial Intelligence, usually refers to narrow AI, which excels at specific tasks but lacks general-thinking capabilities. For example, AI systems can beat grandmasters in chess, recommend products based on user preferences, or detect anomalies in data.
However, these systems cannot transfer their specialized knowledge to different, unrelated tasks.
AGI, on the other hand, can understand, learn, and apply knowledge across various domains, much like a human.
It doesn’t just excel at one task; it can adapt to new tasks and environments without requiring extensive reprogramming.
This versatility is what sets AGI apart from traditional AI systems, aiming for a holistic and integrated approach to intelligence.
While AI has already shown significant successes in specialized areas, AGI aspires to achieve a wider, more general form of intelligence that could revolutionize how we interact with machines and solve complex problems.
Historical Development of AGI
Early Concepts and Theories
Artificial General Intelligence (AGI) dates back to discussions and theories from the mid-20th century. Alan Turing, a British mathematician, proposed in 1950 the idea of a machine that could exhibit intelligent behavior indistinguishable from a human in his seminal paper, “Computing Machinery and Intelligence.”
This led to the creation of the Turing Test, which evaluates a machine’s ability to demonstrate human-like intelligence.
Another key figure, John McCarthy, coined the term “Artificial Intelligence” in 1956 and organized the Dartmouth Conference, where researchers converged to discuss the future of AI, laying foundational ideas for AGI.
Early AI programs like the Logic Theorist (1956) and General Problem Solver (1957) aimed to mimic human problem-solving.
Recent Advancements and Breakthroughs
Recent decades have seen significant strides toward AGI, facilitated by:
- advancements in computational power
- data availability
- machine learning techniques
In the 2010s, deep learning revolutionized the AI field. Models like DeepMind’s AlphaGo demonstrated impressive feats, such as defeating human champions in complex games.
Organizations like OpenAI have made headlines by developing advanced neural network models. GPT-3, for instance, showcases language comprehension and generation abilities, though it remains a form of narrow AI.
Research in neural architecture, transfer learning, and unsupervised learning contributes to AGI progress.
In 2020, the development of neural network frameworks capable of multi-modal learning hinted at AGI. Future breakthroughs are anticipated from ongoing projects focused on integrating different AI techniques to achieve a versatile, human-like intelligence.
Potential Applications of AGI
In Healthcare
Artificial General Intelligence (AGI) can transform healthcare. With its ability to analyze vast datasets, AGI could offer enhanced diagnostic accuracy. For instance, it might quickly identify patterns in medical images, aiding radiologists.
AGI systems could predict patient outcomes by considering various health metrics, potentially reducing hospital readmissions.
Moreover, personalized treatment plans based on a patient’s genetics and lifestyle could become more accessible, optimizing therapeutic outcomes. AGI-enabled robotic surgery might improve precision, leading to fewer complications.
In Automation and Manufacturing
AGI can revolutionize automation and manufacturing. Smart factories driven by AGI could optimize production processes in real-time.
For example, AGI could manage supply chain logistics, reducing downtime and waste.
Predictive maintenance systems powered by AGI might foresee equipment failures before they occur, increasing operational efficiency.
Additionally, adaptive robots using AGI could handle complex tasks and adapt to new ones without human intervention, streamlining production lines.
In Environmental Management
AGI holds potential for environmental management. It could analyze ecological data, offering insights into climate change patterns.
For instance, AGI might model the impact of human activities on ecosystems, guiding conservation efforts.
In agriculture, AGI could optimize resource use, improving crop yields and reducing waste.
Furthermore, AGI could enhance disaster response strategies by predicting natural events like hurricanes or earthquakes, enabling better-preparedness measures.
Challenges and Ethical Considerations
Technical Challenges in Developing AGI
- Developing AGI involves significant technical challenges. Current AI systems, such as deep learning models, excel in specific tasks but lack the ability to generalize across different domains.
- Creating an AGI that can perform a wide range of tasks as humans do necessitates advancements in areas like transfer learning and unsupervised learning.
- Transfer learning is crucial as it allows a model to apply knowledge gained from one task to another, reducing the amount of data required for new tasks.
- Unsupervised learning, where the system learns patterns from data without labeled outcomes, is essential to build more versatile AI systems. Both techniques must mature for AGI to become a reality.
- Scalability is another critical issue. AGI systems require extensive computational resources, from processing power to memory.
For example, training advanced models like GPT-3 involves significant energy and hardware. - Efficient algorithms and hardware acceleration techniques like quantum computing could mitigate these constraints.
- Lastly, ensuring robustness against unpredictable environments is vital. AGI systems must function reliably in diverse and dynamic settings.
- Techniques like reinforcement learning and robust control systems need refinement to handle real-world variability.
Ethical Implications and Societal Impact
The ethical implications and societal impact of AGI are profound. Deployment of AGI could disrupt job markets by automating tasks, leading to massive shifts in employment.
Jobs in sectors like manufacturing and transportation may decline, necessitating workforce re-skilling.
Implementing universal basic income or similar policies could mitigate economic disparities.
Privacy concerns also emerge with AGI. Advanced systems might collect and analyze vast amounts of personal data, raising questions about consent and data security.
Establishing strict data protection regulations and ethical guidelines ensures responsible usage.
Bias in AGI systems is another critical issue. Biased data can amplify societal inequalities and discrimination.
Developers must implement fairness and transparency in AI training processes to avoid perpetuating biases.
Regular audits and adherence to ethical standards help maintain unbiased outcomes.
The potential for AGI to be misused is significant. Scenarios where AGI is employed in surveillance or autonomous weaponry could have catastrophic consequences.
Creating international treaties and robust governance frameworks ensures AGI development aligns with global peace and ethical standards.
Addressing these challenges and ethical considerations requires collaborative efforts from governments, industries, and researchers.
International cooperation and multidisciplinary research pave the way for responsibly harnessing AGI’s potential.
Future Outlook of AGI
Predictions and Trends
Experts foresee significant advancements in AGI over the next few decades, catalyzing global transformation. Current predictions indicate AGI will achieve human-level intelligence within 20 to 50 years.
Trends show increased investment in AGI research, with tech giants like Google and OpenAI leading the charge.
These companies are developing more sophisticated models that can perform various tasks with minimal supervision.
The focus is on creating models that can learn continuously and adapt to new scenarios without extensive reprogramming.
Preparing for an AGI-Enabled World
Governments and organizations are strategizing to brace for an AGI future. Preparing involves updating educational systems to include AI literacy, ensuring a workforce ready for an AI-driven job market.
‘Policymakers are drafting regulations to manage ethical concerns, such as privacy and bias. Collaboration among international entities aims to standardize AGI guidelines, mitigating risks of misuse.
Additionally, industries are investing in upskilling employees to seamlessly integrate AGI technologies, maximizing productivity while minimizing job displacement.
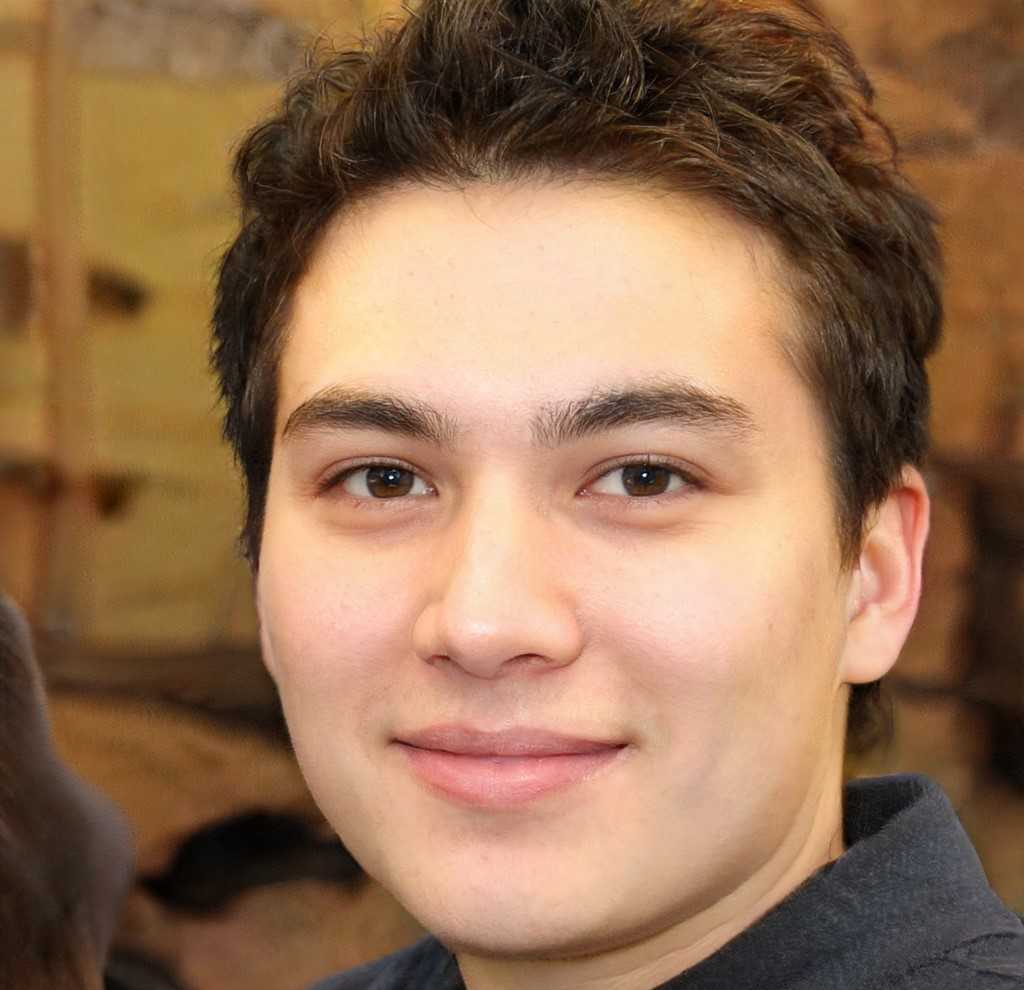
About the author:
Gerthann Stalcupy, the founder of your gtech colony , plays a pivotal role in shaping the direction and content of the platform. As the visionary behind the site. – Learn more