The Importance of Ethics in AI Development
Ethics play a critical role in AI development, guiding how these technologies impact society. As AI systems become more integrated into daily life, ethical considerations ensure their use respects human rights and promotes fairness.
- First, ethical guidelines in AI development help prevent biases.
Biases in algorithms can lead to discriminatory outcomes affecting marginalized groups. By implementing ethical principles, developers can create more inclusive technologies.
For example, machine learning models used in hiring processes should undergo thorough bias testing to avoid unfairly disadvantaging any demographic.
- Second, transparency and accountability form the backbone of ethical AI. Users and developers must understand how AI systems make decisions.
Transparent AI allows for scrutiny, helping identify and rectify errors or unfair practices.
For instance, in healthcare, transparency in AI diagnostics helps doctors trust the technology, benefiting patient care.
- Third, ethical AI development includes respecting user privacy. With AI systems processing massive amounts of data, protecting this information becomes paramount.
Ethical standards should mandate robust data encryption and user consent protocols to prevent misuse.
In the context of law enforcement, ensuring data collected by AI technologies is stored securely and accessed lawfully diminishes privacy invasion risks.
- Fourth, considering long-term societal impacts is essential in ethical AI development. Developers need to assess how AI technologies might affect employment, inequality, and human relations.
For example, automation in industries should be balanced with strategies to mitigate job displacement and improve workforce reskilling opportunities. - Finally, fostering an ethical culture involves continuous learning and adaptation. AI ethics shouldn’t be a one-time check but a continuous process guiding AI through its lifecycle.
Collaboration between ethicists, technologists, and policymakers can better align technological advancements with moral values, ensuring AI contributes positively to society.
By focusing on these ethical dimensions, I aim to contribute to developing AI technologies that are not only innovative but also socially responsible.
Key Ethical Concerns in AI
Privacy and Data Security Issues
AI models depend on vast amounts of data, raising significant privacy concerns.
Ensuring data is collected, stored, and processed securely remains paramount. GDPR and CCPA regulations mandate strict protocols to protect user data. Non-compliance can lead to severe penalties.
Moreover, AI systems can analyze sensitive information, such as:
- health records
- financial details
- personal communications
which necessitates robust encryption and anonymization methods to prevent data breaches.
Bias and Discrimination
Bias in AI systems often results from biased training data.
Algorithms train on datasets that reflect societal prejudices, leading to discriminatory outcomes.
For instance, facial recognition systems may misidentify individuals from certain racial backgrounds due to a lack of diverse training data.
Ensuring fairness requires diverse datasets and regular audits to identify and mitigate bias.
Techniques like fairness-aware machine learning aim to reduce these disparities effectively.
Automation and Job Displacement
AI-driven automation threatens job security in various sectors.
Machines outperform humans in repetitive tasks, resulting in workforce displacement. A 2019 McKinsey report suggests that 45 million Americans might need to switch occupations by 2030 due to automation.
Addressing this issue involves reskilling programs and policies to support workers transitioning to new roles.
Collaboration between industry leaders and policymakers can help mitigate these negative impacts while leveraging AI to create new job opportunities.
Transparency and Accountability
Transparent AI systems build trust among users. Black-box models, where decision-making processes are not understandable, raise concerns.
Ensuring algorithms are interpretable and decisions are traceable is vital. For example, the Explainable AI (XAI) initiative focuses on creating models that offer clear, human-understandable explanations for their outputs.
Accountability measures, including regular audits and compliance with ethical guidelines, ensure responsible AI deployment.
Frameworks for Ethical AI
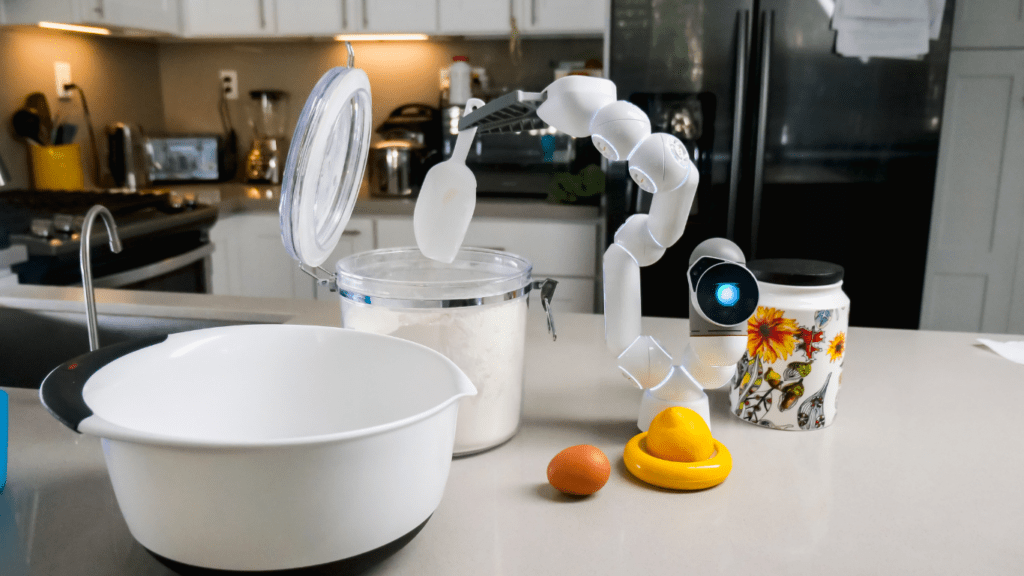
Principles and Guidelines
Ethical AI frameworks rely on well-defined principles and guidelines to ensure responsible development. One widely recognized set is the Asilomar AI Principles, introduced by the Future of Life Institute.
These principles cover crucial areas like:
- transparency
- safety
- shared benefit.
Another key resource is the Ethics Guidelines for Trustworthy AI published by the European Union, emphasizing human agency, privacy, and accountability.
Principles often revolve around fairness and non-discrimination for promoting inclusivity.
For example:
- Microsoft’s Ethical AI principles focus on fairness
- reliability
- privacy
- security
- inclusivity
- transparency
- accountability
Developers can incorporate these principles into AI systems to minimize unintended consequences.
Regulatory and Industry Standards
Regulatory frameworks play a vital role in ensuring AI operates within ethical boundaries.
The General Data Protection Regulation (GDPR) in Europe and the California Consumer Privacy Act (CCPA) in the US set high standards for data protection.
These regulations require organizations to handle personal data responsibly and transparently.
Industry standards also guide ethical AI development. ISO/IEC 24028:2020 provides guidelines on AI risk management, addressing issues like fairness and transparency.
The IEEE Global Initiative on Ethics of Autonomous Systems highlights the importance of ethically aligned design. These standards help organizations align their AI practices with societal expectations.
Specific laws and standards ensure ethical AI aligns with societal values, reducing risks and enhancing trust in AI systems.
Ethical Implementation of AI in Industries
Healthcare
AI implementation in healthcare needs to prioritize patient privacy, data security, and equitable access. Algorithms analyzing patient data must be transparent to ensure accurate diagnostics and treatment plans.
If AI tools identify potential conditions but lack explainability, medical practitioners should intervene to avoid misdiagnoses.
Cases like IBM’s Watson for Oncology show transparency’s importance.
Regulators require adherence to HIPAA for patient data privacy, and implementing fairness-aware machine learning reduces any biases in AI models.
Finance
In finance, AI algorithms detect fraud, predict market trends, and optimize investment strategies. Ethical considerations include ensuring these algorithms don’t introduce biases that could unfairly disadvantage certain groups.
Adhering to regulations like GDPR and CCPA is essential for protecting consumer data. If AI-led trading systems result in volatile markets, oversight mechanisms must be in place to mitigate risks.
Examples from high-frequency trading reveal potential issues with AI systems lacking regulatory checks.
Financial institutions should also implement Explainable AI (XAI) to clarify decision-making processes for compliance and transparency.
Autonomous Vehicles
Safety and accountability are paramount in deploying AI in autonomous vehicles. Ethical dilemmas arise involving decision-making in accident scenarios.
Developers must train AI systems to prioritize human life and minimize harm. Standards like ISO 26262 for automotive safety and guidelines from the IEEE Global Initiative on Ethics of Autonomous Systems are critical for ethical development.
If AI systems malfunction, transparent incident reporting and liability frameworks ensure accountability.
Examples like Tesla’s Autopilot underscore the requirement for continuous monitoring and ethical scrutiny in real-world applications.